在 VTA 上部署预训练的 Darknet 视觉检测模型
导航
在 VTA 上部署预训练的 Darknet 视觉检测模型#
原作者: Hua Jiang
本教程提供了端到端 demo,关于如何在 VTA 加速器设计上运行 Darknet YoloV3-tiny inference 来执行图像检测任务。它展示了 Relay 作为前端编译器,它可以执行量化(VTA 只支持 int8/32 推理)和 graph packing(为了在 core 中支持张量),从而为硬件目标 massage 计算图(compute graph)。
安装依赖#
要在 tvm
中使用 autotvm
包,需要安装一些额外的依赖项。(如果你使用 python2,将 “3” 改为 “2”):
pip3 install "Pillow<7"
带有 Darknet 解析的 YOLO-V3-tiny Model 依赖于 CFFI 和 CV2 库,需要在执行此脚本前安装 CFFI 和 CV2。
pip3 install cffi
pip3 install opencv-python
现在返回 python 代码。导入包。
import sys
import os
import time
import matplotlib.pyplot as plt
import numpy as np
import tvm
import vta
from tvm import rpc, autotvm, relay
from tvm.relay.testing import yolo_detection, darknet
from tvm.relay.testing.darknet import __darknetffi__
from tvm.contrib import graph_executor, utils
from tvm.contrib.download import download_testdata
from vta.testing import simulator
from vta.top import graph_pack
# Make sure that TVM was compiled with RPC=1
assert tvm.runtime.enabled("rpc")
根据 Model 名称 下载 yolo net 配置文件,权重文件,darknet 库文件:
MODEL_NAME = "yolov3-tiny"
REPO_URL = "https://github.com/dmlc/web-data/blob/main/darknet/"
cfg_path = download_testdata(
"https://github.com/pjreddie/darknet/blob/master/cfg/" + MODEL_NAME + ".cfg" + "?raw=true",
MODEL_NAME + ".cfg",
module="darknet",
)
weights_path = download_testdata(
"https://pjreddie.com/media/files/" + MODEL_NAME + ".weights" + "?raw=true",
MODEL_NAME + ".weights",
module="darknet",
)
if sys.platform in ["linux", "linux2"]:
darknet_lib_path = download_testdata(
REPO_URL + "lib/" + "libdarknet2.0.so" + "?raw=true", "libdarknet2.0.so", module="darknet"
)
elif sys.platform == "darwin":
darknet_lib_path = download_testdata(
REPO_URL + "lib_osx/" + "libdarknet_mac2.0.so" + "?raw=true",
"libdarknet_mac2.0.so",
module="darknet",
)
else:
raise NotImplementedError("Darknet lib is not supported on {} platform".format(sys.platform))
下载 yolo 类别和 illustration 前端:
coco_path = download_testdata(
REPO_URL + "data/" + "coco.names" + "?raw=true", "coco.names", module="data"
)
font_path = download_testdata(
REPO_URL + "data/" + "arial.ttf" + "?raw=true", "arial.ttf", module="data"
)
with open(coco_path) as f:
content = f.readlines()
names = [x.strip() for x in content]
定义平台和模型目标#
在 CPU 和VTA 上执行,并定义模型。
# Load VTA parameters from the 3rdparty/vta-hw/config/vta_config.json file
env = vta.get_env()
# Set ``device=arm_cpu`` to run inference on the CPU
# or ``device=vta`` to run inference on the FPGA.
device = "vta"
target = env.target if device == "vta" else env.target_vta_cpu
pack_dict = {
"yolov3-tiny": ["nn.max_pool2d", "cast", 4, 186],
}
# Name of Darknet model to compile
# The ``start_pack`` and ``stop_pack`` labels indicate where
# to start and end the graph packing relay pass: in other words
# where to start and finish offloading to VTA.
# the number 4 indicate the the ``start_pack`` index is 4, the
# number 186 indicate the ``stop_pack index`` is 186, by using
# name and index number, here we can located to correct place
# where to start/end when there are multiple ``nn.max_pool2d``
# or ``cast``, print(mod.astext(show_meta_data=False)) can help
# to find operator name and index information.
assert MODEL_NAME in pack_dict
获取执行远程#
当 target 为 ‘pynq’ 或其他 FPGA 后端时,重新配置 FPGA 和运行时。否则,如果 target 为 ‘sim’,则在本地执行。
if env.TARGET not in ["sim", "tsim"]:
# Get remote from tracker node if environment variable is set.
# To set up the tracker, you'll need to follow the "Auto-tuning
# a convolutional network for VTA" tutorial.
tracker_host = os.environ.get("TVM_TRACKER_HOST", None)
tracker_port = os.environ.get("TVM_TRACKER_PORT", None)
# Otherwise if you have a device you want to program directly from
# the host, make sure you've set the variables below to the IP of
# your board.
device_host = os.environ.get("VTA_RPC_HOST", "192.168.2.99")
device_port = os.environ.get("VTA_RPC_PORT", "9091")
if not tracker_host or not tracker_port:
remote = rpc.connect(device_host, int(device_port))
else:
remote = autotvm.measure.request_remote(
env.TARGET, tracker_host, int(tracker_port), timeout=10000
)
# Reconfigure the JIT runtime and FPGA.
# You can program the FPGA with your own custom bitstream
# by passing the path to the bitstream file instead of None.
reconfig_start = time.time()
vta.reconfig_runtime(remote)
vta.program_fpga(remote, bitstream=None)
reconfig_time = time.time() - reconfig_start
print("Reconfigured FPGA and RPC runtime in {0:.2f}s!".format(reconfig_time))
# In simulation mode, host the RPC server locally.
else:
remote = rpc.LocalSession()
# Get execution context from remote
ctx = remote.ext_dev(0) if device == "vta" else remote.cpu(0)
构建推理 graph executor#
利用 Darknet 库加载下载视觉模型,并用 Relay 编译。编译步骤如下:
从 Darknet 到 Relay 模块的前端翻译。
应用 8 位量化:这里跳过第一个 conv 层和 dense 层,它们都将在 CPU 上的 fp32 中执行。
执行 graph packing 以更改数据布局以进行张量化。
执行常量折叠以减少算子的数量(例如,消除 batch norm 乘法)。
执行 Relay 构建 object 文件。
将 object 文件加载到远程(FPGA 设备)。
生成 graph executor
m
。
# Load pre-configured AutoTVM schedules
with autotvm.tophub.context(target):
net = __darknetffi__.dlopen(darknet_lib_path).load_network(
cfg_path.encode("utf-8"), weights_path.encode("utf-8"), 0
)
dshape = (env.BATCH, net.c, net.h, net.w)
dtype = "float32"
# Measure build start time
build_start = time.time()
# Start front end compilation
mod, params = relay.frontend.from_darknet(net, dtype=dtype, shape=dshape)
if target.device_name == "vta":
# Perform quantization in Relay
# Note: We set opt_level to 3 in order to fold batch norm
with tvm.transform.PassContext(opt_level=3):
with relay.quantize.qconfig(
global_scale=23.0,
skip_conv_layers=[0],
store_lowbit_output=True,
round_for_shift=True,
):
mod = relay.quantize.quantize(mod, params=params)
# Perform graph packing and constant folding for VTA target
mod = graph_pack(
mod["main"],
env.BATCH,
env.BLOCK_OUT,
env.WGT_WIDTH,
start_name=pack_dict[MODEL_NAME][0],
stop_name=pack_dict[MODEL_NAME][1],
start_name_idx=pack_dict[MODEL_NAME][2],
stop_name_idx=pack_dict[MODEL_NAME][3],
)
else:
mod = mod["main"]
# Compile Relay program with AlterOpLayout disabled
with vta.build_config(disabled_pass={"AlterOpLayout", "tir.CommonSubexprElimTIR"}):
lib = relay.build(
mod, target=tvm.target.Target(target, host=env.target_host), params=params
)
# Measure Relay build time
build_time = time.time() - build_start
print(MODEL_NAME + " inference graph built in {0:.2f}s!".format(build_time))
# Send the inference library over to the remote RPC server
temp = utils.tempdir()
lib.export_library(temp.relpath("graphlib.tar"))
remote.upload(temp.relpath("graphlib.tar"))
lib = remote.load_module("graphlib.tar")
# Graph executor
m = graph_executor.GraphModule(lib["default"](ctx))
yolov3-tiny inference graph built in 24.58s!
/media/pc/data/4tb/lxw/anaconda3/envs/torch/lib/python3.10/site-packages/tvm/driver/build_module.py:267: UserWarning: target_host parameter is going to be deprecated. Please pass in tvm.target.Target(target, host=target_host) instead.
warnings.warn(
One or more operators have not been tuned. Please tune your model for better performance. Use DEBUG logging level to see more details.
[15:39:08] /media/pc/data/4tb/lxw/books/tvm/src/tir/transforms/arg_binder.cc:95: Warning: Trying to bind buffer to another one with lower alignment requirement required_alignment=256, provided_alignment=128
[15:39:08] /media/pc/data/4tb/lxw/books/tvm/src/tir/transforms/arg_binder.cc:95: Warning: Trying to bind buffer to another one with lower alignment requirement required_alignment=256, provided_alignment=128
[15:39:09] /media/pc/data/4tb/lxw/books/tvm/src/tir/transforms/arg_binder.cc:95: Warning: Trying to bind buffer to another one with lower alignment requirement required_alignment=256, provided_alignment=128
[15:39:09] /media/pc/data/4tb/lxw/books/tvm/src/tir/transforms/arg_binder.cc:95: Warning: Trying to bind buffer to another one with lower alignment requirement required_alignment=256, provided_alignment=128
[15:39:09] /media/pc/data/4tb/lxw/books/tvm/src/tir/transforms/arg_binder.cc:95: Warning: Trying to bind buffer to another one with lower alignment requirement required_alignment=256, provided_alignment=128
[15:39:10] /media/pc/data/4tb/lxw/books/tvm/src/tir/transforms/arg_binder.cc:95: Warning: Trying to bind buffer to another one with lower alignment requirement required_alignment=256, provided_alignment=128
[15:39:10] /media/pc/data/4tb/lxw/books/tvm/src/tir/transforms/arg_binder.cc:95: Warning: Trying to bind buffer to another one with lower alignment requirement required_alignment=256, provided_alignment=128
[15:39:10] /media/pc/data/4tb/lxw/books/tvm/src/tir/transforms/arg_binder.cc:95: Warning: Trying to bind buffer to another one with lower alignment requirement required_alignment=256, provided_alignment=128
[15:39:11] /media/pc/data/4tb/lxw/books/tvm/src/tir/transforms/arg_binder.cc:95: Warning: Trying to bind buffer to another one with lower alignment requirement required_alignment=256, provided_alignment=128
[15:39:11] /media/pc/data/4tb/lxw/books/tvm/src/tir/transforms/arg_binder.cc:95: Warning: Trying to bind buffer to another one with lower alignment requirement required_alignment=256, provided_alignment=128
[15:39:11] /media/pc/data/4tb/lxw/books/tvm/src/tir/transforms/arg_binder.cc:95: Warning: Trying to bind buffer to another one with lower alignment requirement required_alignment=256, provided_alignment=128
2022-07-02 15:39:15.872 INFO load_module /tmp/tmpx4fzz6vr/graphlib.tar
执行图像检测推理#
检测已下载的图像:
[neth, netw] = dshape[2:]
test_image = "person.jpg"
img_url = REPO_URL + "data/" + test_image + "?raw=true"
img_path = download_testdata(img_url, test_image, "data")
data = darknet.load_image(img_path, neth, netw).transpose(1, 2, 0)
# Prepare test image for inference
plt.imshow(data)
plt.show()
data = data.transpose((2, 0, 1))
data = data[np.newaxis, :]
data = np.repeat(data, env.BATCH, axis=0)
# Set the network parameters and inputs
m.set_input("data", data)
# Perform inference and gather execution statistics
# More on: :py:method:`tvm.runtime.Module.time_evaluator`
num = 4 # number of times we run module for a single measurement
rep = 3 # number of measurements (we derive std dev from this)
timer = m.module.time_evaluator("run", ctx, number=num, repeat=rep)
if env.TARGET in ["sim", "tsim"]:
simulator.clear_stats()
timer()
sim_stats = simulator.stats()
print("\nExecution statistics:")
for k, v in sim_stats.items():
# Since we execute the workload many times, we need to normalize stats
# Note that there is always one warm up run
# Therefore we divide the overall stats by (num * rep + 1)
print("\t{:<16}: {:>16}".format(k, v // (num * rep + 1)))
else:
tcost = timer()
std = np.std(tcost.results) * 1000
mean = tcost.mean * 1000
print("\nPerformed inference in %.2fms (std = %.2f) for %d samples" % (mean, std, env.BATCH))
print("Average per sample inference time: %.2fms" % (mean / env.BATCH))
# Get detection results from out
thresh = 0.5
nms_thresh = 0.45
tvm_out = []
for i in range(2):
layer_out = {}
layer_out["type"] = "Yolo"
# Get the yolo layer attributes (n, out_c, out_h, out_w, classes, total)
layer_attr = m.get_output(i * 4 + 3).numpy()
layer_out["biases"] = m.get_output(i * 4 + 2).numpy()
layer_out["mask"] = m.get_output(i * 4 + 1).numpy()
out_shape = (layer_attr[0], layer_attr[1] // layer_attr[0], layer_attr[2], layer_attr[3])
layer_out["output"] = m.get_output(i * 4).numpy().reshape(out_shape)
layer_out["classes"] = layer_attr[4]
tvm_out.append(layer_out)
thresh = 0.560
# Show detection results
img = darknet.load_image_color(img_path)
_, im_h, im_w = img.shape
dets = yolo_detection.fill_network_boxes((netw, neth), (im_w, im_h), thresh, 1, tvm_out)
last_layer = net.layers[net.n - 1]
yolo_detection.do_nms_sort(dets, last_layer.classes, nms_thresh)
yolo_detection.draw_detections(font_path, img, dets, thresh, names, last_layer.classes)
plt.imshow(img.transpose(1, 2, 0))
plt.show()
RemoteDisconnected('Remote end closed connection without response')
Download attempt 0/3 failed, retrying.
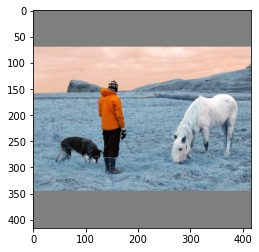
Execution statistics:
inp_load_nbytes : 25462784
wgt_load_nbytes : 17558016
acc_load_nbytes : 96128
uop_load_nbytes : 5024
out_store_nbytes: 3396224
gemm_counter : 10578048
alu_counter : 849056
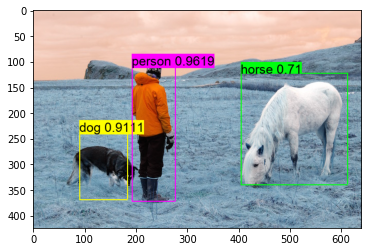